Ever since Google announced that it would be releasing an open-source Media Mix Modelling (MMM) package, which adds to and improves upon its predecessor, LightweightMMM, data scientists and marketers have been eagerly waiting for access to the platform. Most were left waiting until Google announced they would release it to the world on the 29th of January 2025.
What did we already know?
In January, just before the spontaneous release of Google Meridian, we published a blog outlining everything Meridian. Our conclusion at the time was that, while Meridian provides a platform built on similar foundations to current MMM tools, it lacked the flexibility we needed at Impression. Based on what we knew then, we felt Meridian didn’t support features like channel hierarchical modelling and time-variant media channels, which we could implement using PyMC. The ability to customise models fully, in our opinion, is vital in building an MMM, because every business is different. Despite this, we must constantly try to improve our services, and if Meridian is the answer to that, we would be missing an opportunity if we dismissed it before it is even released!
What Google Meridian gets right
A fully functional Bayesian MMM Model
Out of the box, Meridian includes:
- Bayesian inference for uncertainty estimation.
- Adstock transformation to model carryover effects.
- Saturation functions to capture diminishing returns.
- Hierarchical geo-modelling, which is useful for regional insights.
It’s a solid implementation of modern MMM best practices.
Built-in priors reduce the learning curve
One advantage of Meridian is that Google has pre-configured priors and hyperparameters, parameters that control the learning process of a model, based on industry knowledge. This means you don’t have to spend as much time tuning the model, which is a plus if you’re not familiar with Bayesian statistics.
Effective visual reporting
After running your MMM, Meridian has the option to output all of your data into a two-page HTML report. In my opinion, this report is an excellent way to present your model outputs, offering immediate insights into channel performance and ROI. The waterfall chart (see below) effectively breaks down sales contributions, while the response curves provide a visual understanding of saturation points and diminishing returns for each paid media channel. This allows for quick identification of high-performing areas and potential optimisation opportunities.
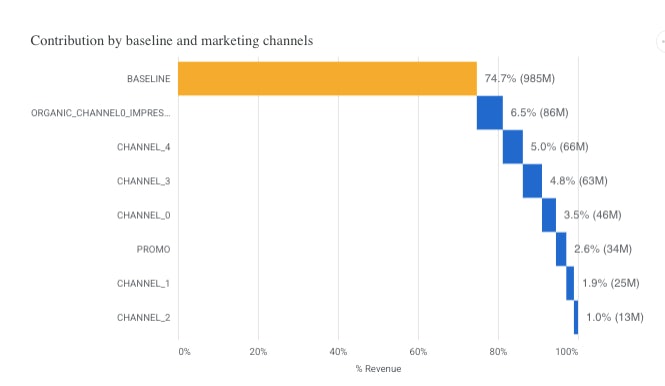
But here’s the problem: MMM is not a one-size-fits-all problem. And that’s exactly where Meridian falls short.
What Meridian could improve
Meridian lacks flexibility for advanced users
Meridian provides a solid foundation for Marketing Mix Modelling, but it does have some limitations when it comes to customisation, especially from the perspective of marketing data scientists.
If you need to use different adstock or saturation functions, you’re limited to what Meridian offers. Custom likelihood functions aren’t easily adjustable, and if your business has unique constraints, making the necessary changes could be a challenge.
MMM isn’t a one-size-fits-all approach. Different industries, marketing channels, and regions require different modelling choices, and Meridian’s structured framework might not work for everyone. For example, the figure below shows the built-in adstock effect (in blue), but Meridian doesn’t support the delayed adstock effect (in orange), which is useful for businesses with longer customer decision cycles, like buying a car or planning a wedding.
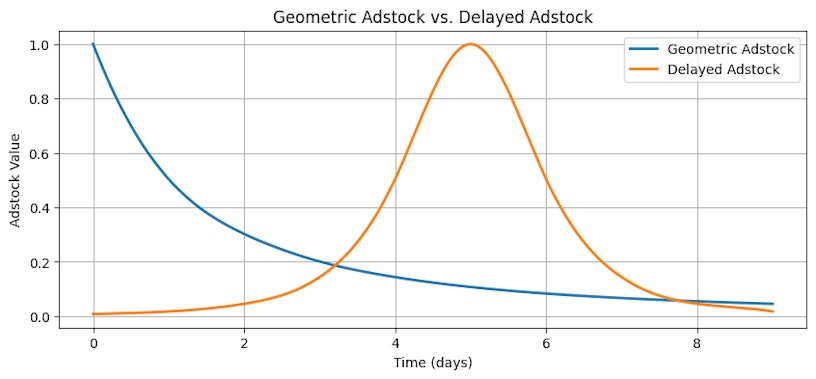
Marketing Mix Modelling will always require expertise
One of Google’s main selling points is that Meridian makes MMM more accessible. But the reality is:
- Model selection and validation require deep statistical expertise. Even with built-in priors, interpreting results and making adjustments still require knowledge of Bayesian statistics.
- Business context cannot be automated. A pre-built model, no matter how well-designed, will never fully capture the nuances of every business’s marketing reality.
- Debugging Bayesian models is not always easy. Even in structured frameworks like Meridian, properly diagnosing model fit issues or adjusting priors requires technical skill.
So, who is Meridian really for? If a company has the expertise to run MMM at a high level, it may still prefer a more flexible, customisable solution. But for businesses looking for an easier entry point into MMM, Meridian provides a structured approach with industry best practices.

At its core, MMM is not a problem that can be fully automated. Every business has unique challenges, and pre-built solutions like Meridian and Robyn, Meta’s open-source MMM, will always have some limitations.
For teams that prioritise full control, customisation, and advanced modelling techniques, PyMC remains our preferred choice. It allows for:
- Defining custom priors, likelihoods, and transformations based on business needs.
- Adapting the model for any industry, geography, or business constraint.
- Scaling efficiently with modern Bayesian inference techniques.
However, for teams that want a more standardised approach with less manual tuning, Meridian offers practical alternatives. The key is understanding your business needs and selecting the right tool accordingly.
At the end of the day, Marketing Mix Modelling is about understanding marketing effectiveness at a deep level, and that requires careful model selection, validation, and interpretation, regardless of the platform used.