Measuring media effectiveness and optimising budget allocation is a challenge for any marketer. As we constantly seek better ways to ensure that every penny spent contributes to the overall success of our campaigns, the traditional approach of relying on heuristics, which involves using rule-of-thumb methods, often falls short in providing actionable insights for long-term success.
Additionally, over the last couple of decades, there has been an over-reliance on digital Multi-Touch Attribution (MTA) models. These attribution models track and assign credit to multiple media channels based on “touchpoints” within the customer journey. However, Multi-Touch Attribution relies on its ability to see each step of the customer journey. Although, in recent years, this has become increasingly difficult due to the decaying lifetime of the cookie as a result of the Intelligent Tracking Prevention (ITP) browser feature introduction in 2017.
To address this challenge, marketers need to use more advanced approaches in producing data-driven insights in order to enhance their decision-making processes.
Welcome to the world of Media Mix Modelling, or Marketing Mix Modelling – the secret weapon for optimising marketing strategies.
What is Media Mix Modelling?
Media mix modelling is a powerful statistical tool used by marketers and media scientists to analyse, optimise and forecast advertising strategies.
Media mix modelling helps you to determine how different media channels contribute towards achieving the desired marketing goals and uses this to provide an optimal budget distribution. It works by analysing historical data on various media channels and their impact on KPIs. It utilises collected data on media spend, KPIs such as conversions and other context variables such as events, trends or seasonality.
What can Media mix modelling do for you?
Media mix modelling allows you to fully understand how effective each media channel is and to what extent each channel contributes towards meeting KPIs such as revenue, enquiries or other outcomes.
This is done by taking into account not only the effects of each media channel but also how any context variables such as sales events, seasonality or holidays impact KPIs. This provides clarity on which variables are actually impacting KPIs and allows us to quantify their impact. For instance, is a peak or trough in revenue caused by a change in media investment or something else? Media mix modelling incorporates these context variables to allow for a better explanation of anomalies captured within the data set.
We utilise the Bayesian framework to perform media mix modelling. This allows for any prior knowledge or beliefs that may have to be included in the modelling process for a better quality of model refinement. For instance, if a particular media channel is known (through testing) to have short-term effects that quickly decay over time then it will be beneficial to include this knowledge in the model before incorporating the data.
Besides strengthening the model through the use of prior knowledge or “priors”, Bayesian models provide a way to account for any uncertainty within the decision-making process. For instance, say the media mix model provides predictions for a particular channel, attached to these predictions will be corresponding probabilities and in most cases, the prediction of the greatest probability will be used.
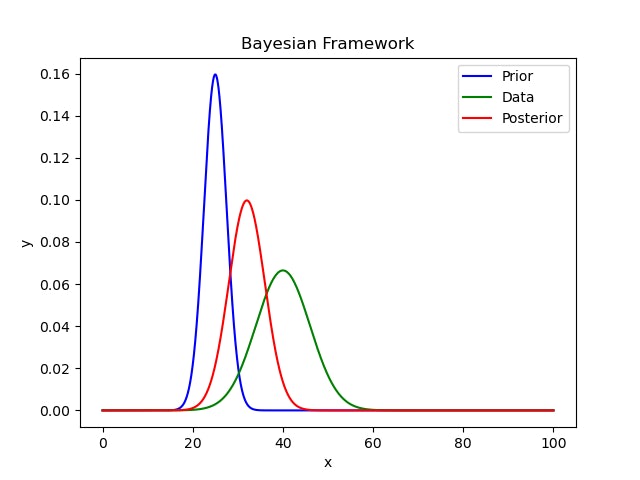
Generally, Bayesian models use a combination of prior knowledge and the data to produce what’s called the posterior distribution. It is this posterior distribution that contains the results and the corresponding uncertainties you require.
Once a media mix model has been fitted with all the relevant variables and priors included, you can then also use the media mix model to run simulations on budget allocation among channels, and forecast with different budget scenarios. Media mix modelling allows you to determine the nature of how each media channel affects KPIs, the saturation effects of each media channel and the relative adstock effects.
Advertising adstock, also known as advertising carry-over, refers to the prolonged effect of advertising after exposure and is best described as the residual effects of an advert that stays with people even after they’ve seen it. Media mix modelling uses various sub-models to best mimic the effects of adstock per channel using a combination of prior knowledge and the data.
Saturation, on the other hand, occurs when a consumer has been exposed to an advert so frequently that it no longer grabs their attention or influences behaviour. When saturation happens, the effects of the advert diminish over time. In the context of media mix modelling, saturation occurs when further spend on a particular channel begins to generate a non-increasing return on ad spend. Similar to adstock effects, media mix modelling uses various sub-models to best mimic the effects of saturation per channel using a combination of prior knowledge and the data.
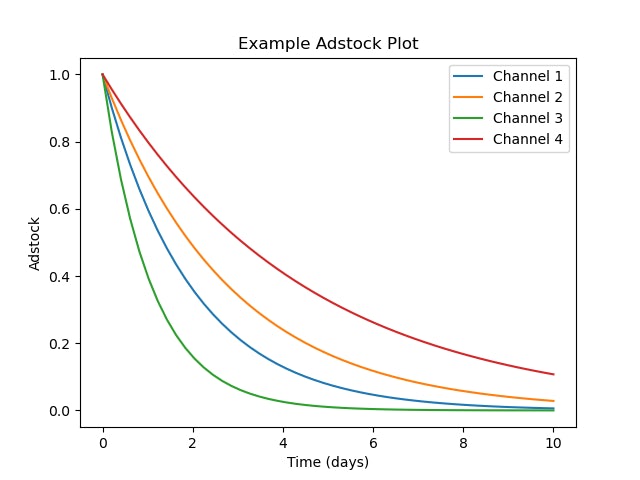
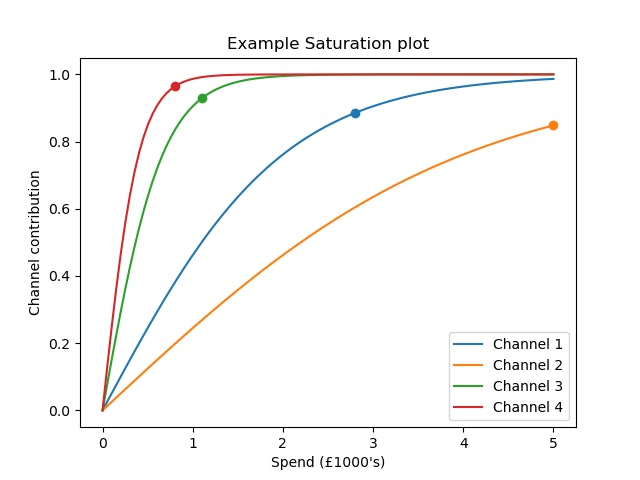
The above images are plots of example adstock and saturation models for four different media channels. The point shown on the saturation plot denotes the saturation point.
Once determined from the model, a new budget allocation is then proposed based on all these factors. Once budget allocation has been performed, the predicted return on ad spend (ROAS) for each channel can be derived.
Overall, media mix modelling provides statistically significant insights into media investment which ensures you aren’t over/under spending on certain media channels. In addition to allowing you to understand anomalies in your KPIs, media mix modelling provides insights concerning how best to avoid or exploit these anomalies with regard to media investment.
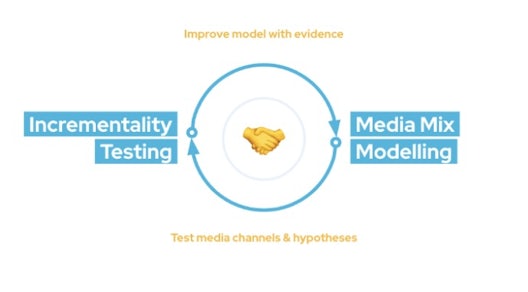
Incrementality studies can also be used to inform media mix modelling by providing useful insights into the incremental impact of various marketing channels. These studies provide prior insights into how a particular media channel contributes to KPI’s in comparison to a scenario with no marketing activity. The model then uses a combination of the data and prior knowledge to assess and forecast business performance.
Additionally, the model enables you to fine-tune to what extent prior knowledge influences results, therefore if there is a lot of prior uncertainty around the nature of a particular media channel you can incorporate this lack of information into the model. Once any prior knowledge/beliefs have been integrated into the model, the data is then used to update this knowledge to provide useful insights derived from both a combination of the data and prior knowledge.
Once a media mix mode is fitted, you can use this model to predict future KPIs based on either the current or the new optimised budget. Over time these predictions can be compared with reality and as a result, your understanding of the data and modelling process can be iteratively improved over time.
Attribution modelling
Attribution refers to a method that identifies which marketing activities contribute to the KPI of concern. For example, say a customer saw an ad on Facebook, clicked on the website, received a follow-up email then decided to purchase a product, where does the credit for the purchase go? Should the credit go directly to the email (the last touch point) or should it be distributed across each channel (data-driven attribution)? Attribution models aim to accurately capture and model the customer journey and provide insights on channel contribution accordingly.
With many platforms phasing out the cookie, a different approach for assessing channel contribution on aggregate is needed and this is where marketing mix modelling is best suited.
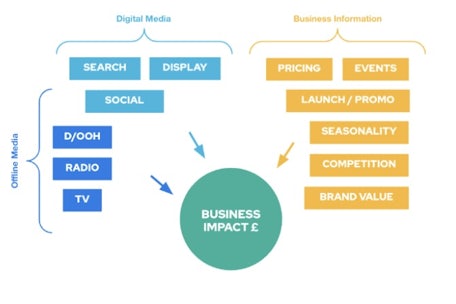
Advantages of Media Mix Modelling
It incorporates prior business knowledge
- Incorporating business insights such as marketing trends, pricing information or competitor activities the modelling process can be tailored towards the unique context of the business in question. This ensures recommendations derived from the media mix model align with the goals and objectives of the organisation.
Includes offline media contribution
- The effects of offline media are often tricky to include when assigning credit or contribution to media channels, especially in the case of multi-touch attribution models. Given data regarding offline media spend and performance, media mix modelling can provide data-driven insights into the effects of offline media and include offline media in the new budget allocation.
Data-driven decision making
- Media mix modelling allows you to quantify the impact generated by various different media channels and uses this to provide data-driven insights into budget allocation and marketing strategies.
Maximises ROAS
- Obtains an optimal media mix and forecasts channel contribution to maximise ROAS across the entire media channel portfolio.
Avoids being misled by other variables
- Any peaks or troughs in the data can be accounted for by including non-media variables in the modelling process.
Uses the most up to date software to aid with modelling
- Here at Impression, we use the most up-to-date and statistically advanced software packages to quantify channel contribution, perform budget allocation and optimise ROAS.
Limitation of Media Mix Modelling
Data limitations
- Typically 2-3 years of data is required to run an accurate media mix model. This is because the model will struggle to truly capture the effects of seasonality with limited data. Additionally, the data must be of good quality as incomplete or inconsistent data can limit the accuracy of the results.
How is Media Mix Modelling done?
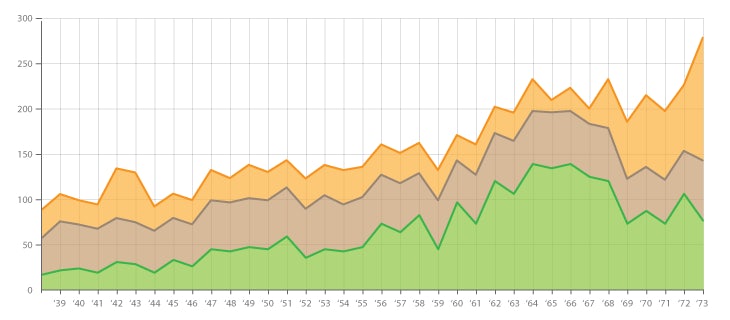
The first step to fitting a Media Mix Modelling is data collection and preparation. Data including variables such as marketing events, spend data, sales data and other external factors including seasonality and holidays is required. This data should be cleansed and organised in a structured manner for model input.
Secondly the relevant spend and context variables are then transformed in order to take into account the effects of adstock and saturation. The nature of these transformations can be tuned according to any prior beliefs/knowledge one may have. Although the order of these transformations does matter, in general practice the adstock transformation is applied and then the saturation transformation afterwards.
Once the data has been transformed a statistical model is then fitted with aim of mimicking KPIs. The modelling process involves advanced statistical and machine learning techniques depending on the context of the analysis. This model is used to estimate the impact generated by various media channels.
Once an optimal model has been defined, it can be used to assess the impact of different budget strategies. Through estimation of the optimal media mix, an optimal budget allocation can be derived which identifies the most effective way to maximise the ROAS. Any uncertainty associated with such predictions can also be extracted from the model, which provides clarity regarding the validity of the results. The larger variation in current media spend and the further we extrapolate from current media spend the larger any associated errors become.
Lastly, model refinement over time is very important. This may involve updating the data, modifying prior beliefs or adding new variables to account for new abnormalities in the data. Keeping the data up-to-date and adapting the model over time will only improve the predictive validity of the model.
Media mix modelling is a valuable tool that allows marketers to optimise their marketing strategies. Through the analysis of data and understanding of how different media channels contribute towards KPIs, businesses can make informed decisions on resource allocation. Media mix modelling helps to maximise channel contribution which leads to better results and a stronger return on investment.
If you’re looking to improve KPIs and overall business performance, based on data-driven statistical evidence, media mix modelling is the way forward!
For more insights and guides on measurement and GA4, be sure to take a look at our blog. If you want to talk about your business aspirations, get in touch!